A team from the University of Maryland has developed COLORBENCH, the first dedicated benchmark for systematically evaluating how vision-language models (VLMs) perceive and process color.
According to the researchers, the results reveal fundamental weaknesses in color perception—even among the largest models currently available.
Color plays a central role in human visual cognition and is critical in fields such as medical imaging, remote sensing, and product recognition. However, it remains unclear whether VLMs interpret and use color in comparable ways.
COLORBENCH assesses models across three main dimensions: color perception, color reasoning, and robustness to color alterations. The benchmark includes 11 tasks with a total of 1,448 instances and 5,814 image-text queries. Tasks require models to recognize colors, estimate color proportions, count objects with specific colors, or resist common color illusions. In one test, for example, models are evaluated for consistency when specific image segments are rotated through different colors.
Ad
THE DECODER Newsletter
The most important AI news straight to your inbox.
✓ Weekly
✓ Free
✓ Cancel at any time
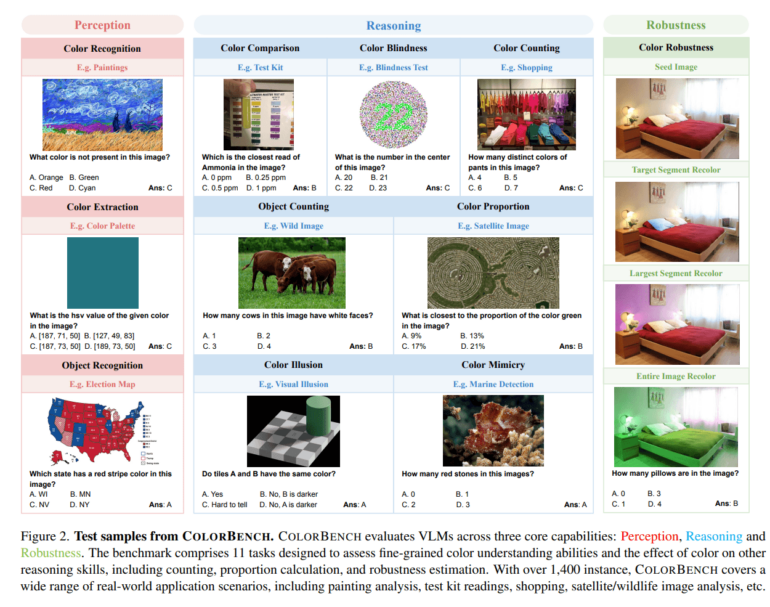
Larger models perform better—but not by much
The benchmark was used to test 32 widely used VLMs, such as GPT-4o, Gemini 2, and a range of open-source models with up to 78 billion parameters. Results show that larger models generally perform better, but the effect is less pronounced than in other benchmarks. The performance gap between open-source and proprietary models is also relatively small.
All tested models showed particularly weak performance on tasks like color counting or color blindness tests, often scoring below 30% accuracy. Even in color extraction tasks—where models are asked to identify specific HSV or RGB values—large models typically achieved only moderate scores. They performed better in tasks involving object or color recognition, which the researchers attribute to the nature of the training data.
Color can mislead models
One key finding is that while VLMs often rely on color cues, these signals can sometimes lead to incorrect conclusions. In tasks involving color illusions or camouflaged object detection, model performance improved when the images were converted to grayscale—suggesting that color information was more misleading than helpful in those cases. Conversely, some tasks could not be completed meaningfully without color.
The study also found that chain-of-thought (CoT) reasoning increased not only performance on reasoning tasks but also robustness to color changes—even though only the image colors, not the questions, were altered. With CoT prompting, for instance, GPT-4o's robustness score rose from 46.2% to 69.9%.
Limited scaling of vision encoders
The researchers observed that model performance correlated more strongly with the size of the language model than with the vision encoder. Most vision encoders remain relatively small—typically around 300 to 400 million parameters—limiting the ability to assess their role in color understanding. The team identifies this as a structural limitation in current VLM design and recommends further development of visual components.
Recommendation
COLORBENCH is publicly available and intended to support the development of more color-sensitive and robust vision-language systems. Future versions of the benchmark are expected to include tasks that combine color with texture, shape, and spatial relationships.